Editor’s Note: The SCM thesis Machine Learning and Optimization-Based Modeling for Asset Management was authored by Carlos Rafavy and Justin Casey and supervised by Dr. Andrés Felipe Muñoz-Villamizar. For more information on the research, please contact Dr. Josué Velázquez Martínez ([email protected]), Executive Director, MIT SCM.
Our sponsoring company is a supplier of pumping equipment with branches across the United States. Its products, specifically in the rental segment, are general purpose dewatering pumps designed for use in the construction, municipal, industrial, and emergency response industries.
The company redistributes dewatering pumps from branch to branch weekly to better position the equipment for rent. With millions of dollars spent to redistribute this equipment,, the company is looking for ways to improve asset utilization by ensuring that the right types of pumps are available when required, without impeding the mobility of the units.
To solve this problem, we took two approaches: develop a sophisticated demand forecast model and an optimization model.
Shifting from instinct to data-driven decisions
Historically, the company relied mostly on intuition to make allocation decisions. Absent from the decision-making process was the use of a statistical demand forecast or mathematical optimization model. We set out to change this situation by developing an integrated decision tool.
Two standard processes drove our research in operations: demand forecasting and transportation optimization. We wanted to develop a model to accurately predict demand, which would then serve as an input to the optimization model. The objective of the optimization model was to reduce the distance traveled. By predicting demand at each branch, our sponsor can proactively position their pumps to meet customer needs. At the same time, the optimization model will help to minimize transportation costs.
After researching the industrial equipment rental and water infrastructure industries as well as different machine learning and mathematical optimization techniques, we selected one machine learning algorithm out of four choices for the demand forecast. The primary constraints of the optimization model are related to demand and supply at each branch.
A two-model solution
During the initial phases of the project, we learned about the distribution patterns and intermittent demand nature of the company’s products. To solve this problem, we created several time-series features. After various iterations of each predictive algorithm, we selected a feed-forward neural network (FNN) for demand forecasting. The final step of our project was to incorporate the predictions of the FNN model in the optimization model.
We believe both of our models, run jointly, will help solve the company’s problem. The forecasting model will provide the company with an accurate prediction of their demand. The optimization model will reposition assets most efficiently by reducing transportation miles.
The simultaneous use of each of the models will increase asset utilization and minimize inefficient equipment transfers from branch to branch. Previously, the company had no tools for predicting demand and transporting products. Now, the enterprise has an integrated decision tool, run each month to assist with asset allocation decisions.
Every year, approximately 80 students in the MIT Center for Transportation & Logistics’s (MIT CTL) Master of Supply Chain Management (SCM) program complete approximately 45 one-year research projects.
These students are early-career business professionals from multiple countries, with two to 10 years of experience in the industry. Most of the research projects are chosen, sponsored by, and carried out in collaboration with multinational corporations. Joint teams that include MIT SCM students and MIT CTL faculty work on real-world problems. In this series, they summarize a selection of the latest SCM research.
SC
MR
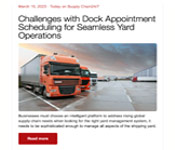
Latest Supply Chain News
- April manufacturing output slides after growing in March
- Q1 sees a solid finish with positive U.S.-bound import growth, notes S&P Global Market Intelligence
- World Trade Centers offers a helping hand to create resilient, interconnected supply chains
- 6 Questions With … Sandeep Bhide
- MIT CTL offering humanitarian logistics course
- More News
Latest Podcast
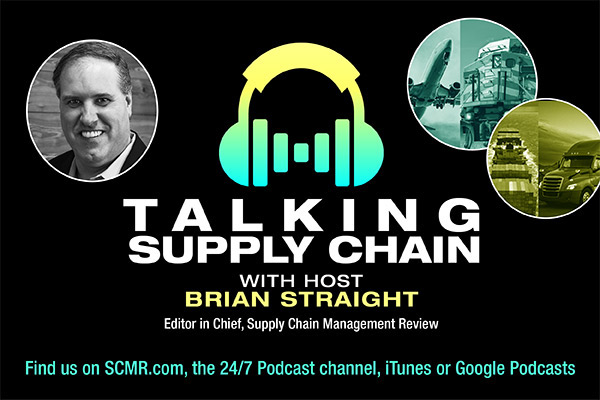
Explore
Business Management News
- April manufacturing output slides after growing in March
- Q1 sees a solid finish with positive U.S.-bound import growth, notes S&P Global Market Intelligence
- 6 Questions With … Sandeep Bhide
- MIT CTL offering humanitarian logistics course
- Bridging the ESG gap in supply chain management: From ambition to action
- Few executives believe their supply chains can respond quickly to disruptions
- More Business Management
Latest Business Management Resources
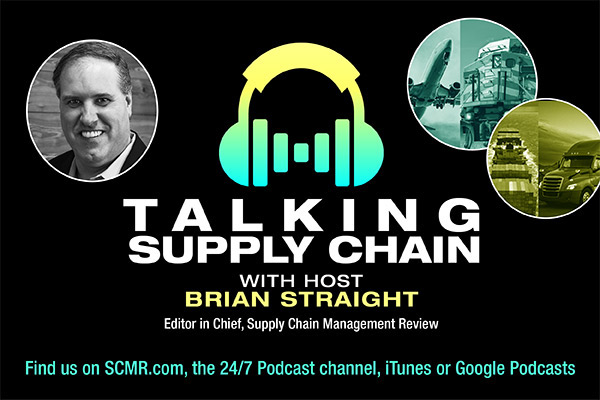
Subscribe
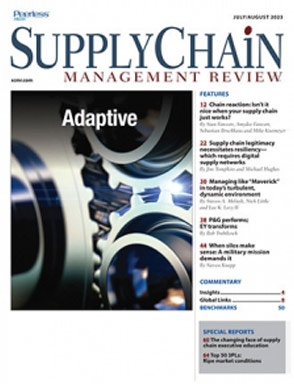
Supply Chain Management Review delivers the best industry content.
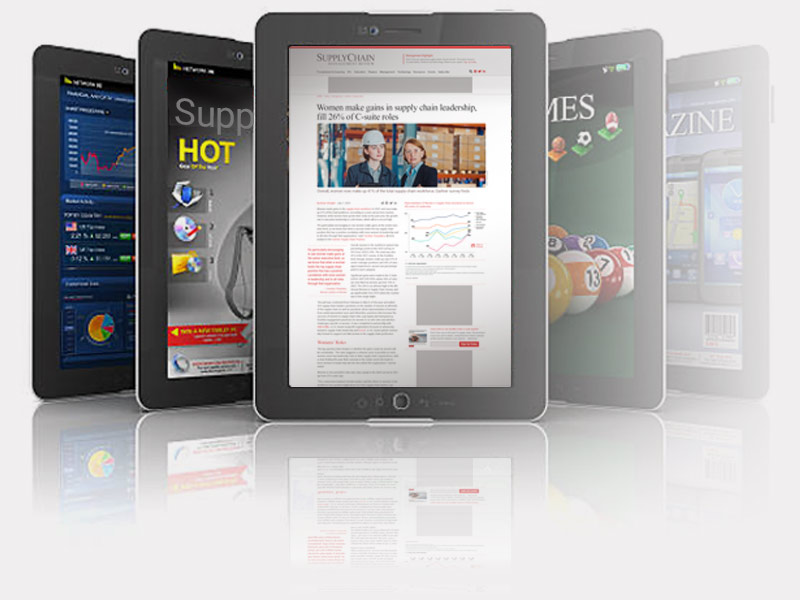
Editors’ Picks
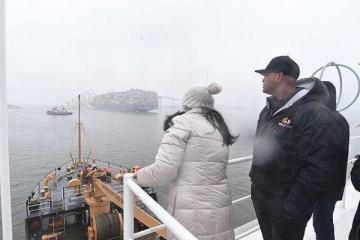

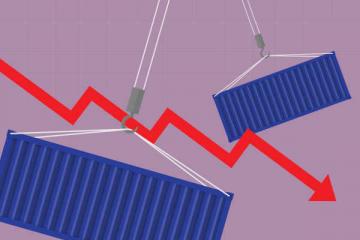
