Editor’s Note: Every year, 40 or so students in the MIT Center for Transportation & Logistics' (MIT CTL) Master of Supply Chain Management (SCM) program complete one-year research projects. The students are early-career business professionals from multiple countries with 2 to 10 years of experience in the industry. Most of the research projects are chosen, sponsored by, and carried out in collaboration with multinational corporations. Joint teams that include MIT SCM students and MIT CTL faculty work on the real-world problems. In this series, we summarize a selection of the latest SCM research. The SCM research project Enhancing S&OP Performance with Analytics was authored by Minhaaj Khan and Srideepti Kidambi, and supervised by Dr. Tugba Efendigil, Research Scientist, MIT Center for Transportation & Logistics. For more information on the research please contact Tugba Efendigil at [email protected].
Businesses implement the Sales and Operations Planning (S&OP) process to focus, align and synchronize all the different functions of their organizations. Given the significance of this effort, it's surprising that most businesses don't carefully consider the risks in their S&OP forecast. As a result, many businesses are run on suboptimal plans that drive up supply chain costs and eat into their bottom lines.
This was the problem addressed in a capstone research project sponsored by a company in the CPG industry that sells health and nutrition products. Working with contract manufacturers, the sponsor company had to plan production three months ahead of forecasted demand. While holding extra inventory was one of the company's solutions to address this reality, the short shelf-life of their products lead to high obsolescence. Its inability to assess risks in the S&OP plan meant that the CPG company struggled to balance supply and demand.
Data, Data, Everywhere
A combination of objective (i.e. demand history, statistical forecast) and subjective (i.e. management input) data was used to identify the risk patterns in the sponsor company's S&OP data. We focused on the risks of over-forecast, under-forecast, the use of safety stock and stock outs. These risks were analyzed for one of the company's smaller brands, which was struggling with mismatches in supply and demand. Using predictive analytics, we hunted for patterns that would help predict these mismatches and reduce S&OP risks.
Applying the data mining technique of supervised learning, we used historical S&OP data where we identified risks (e.g. 50% over forecast) based on known company history. We fed this historical data into supervised learning algorithms. These algorithms “learned” how to predict the risks and were applied to future S&OP plans where the risks were unknown and had to be determined. For example, to determine over-forecast risk demand patterns, inventory, production and consensus forecast data were our model inputs. Despite lacking promotion data – a key driver of supply/demand imbalance – the model accurately predicted over-forecast risks with high accuracy.
More Money, Less Problems
We applied our model to determine a 50% over-forecast risk for the small brand we focused on in the study. Where the model predicted that over-forecast risk was highly probable in the S&OP forecast, the forecast was reduced by 50%. Applying this model increased the profit margin for this brand by 3.8% over a three-month period. Extrapolating our methodology to all the company's brands could help it increase gross profit by $17MM annually.
This study provides a glimpse of the potential of predictive analytics to successfully capture business risks in the S&OP plan. The results were achieved even though key predictors were missing and we worked without big data.
Our findings should encourage companies that are hesitant to pursue predictive analytics to begin the search for value in their data – even if their data doesn't fit the big data mold. Companies that improve the quality of their data and acquire the knowledge necessary to leverage predictive analytics can better plan for what to make, when, and for whom, to drive large increases in profit and gain significant competitive advantage.
In other words, when addressing the problem of S&OP forecast risk, a key issue is not what your data can do for you, but what you can do with your data.
SC
MR
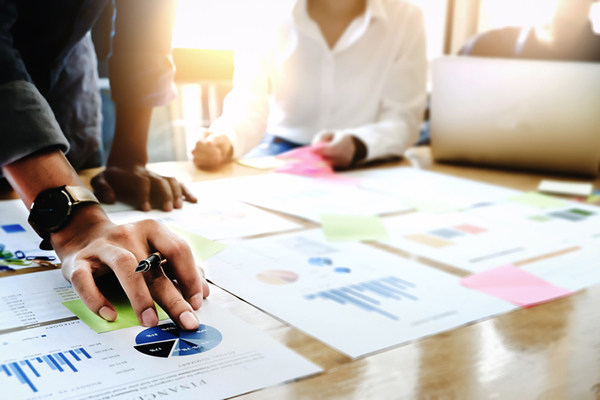
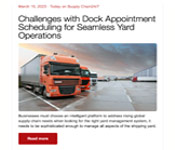
Latest Supply Chain News
Latest Podcast

Explore
Topics
Education News
- Do net-zero goals matter?
- Leadership development for supply chain leaders
- ASCM introduces Supplier Relationship Management certificate
- An educated workforce is loyal, but what type of education is best?
- When the scales tilt: Making vaccine access work for all
- Five organizational action areas for developing supply chain talent
- More Education
Latest Education Resources

Subscribe
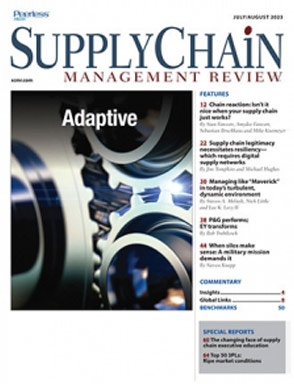
Supply Chain Management Review delivers the best industry content.
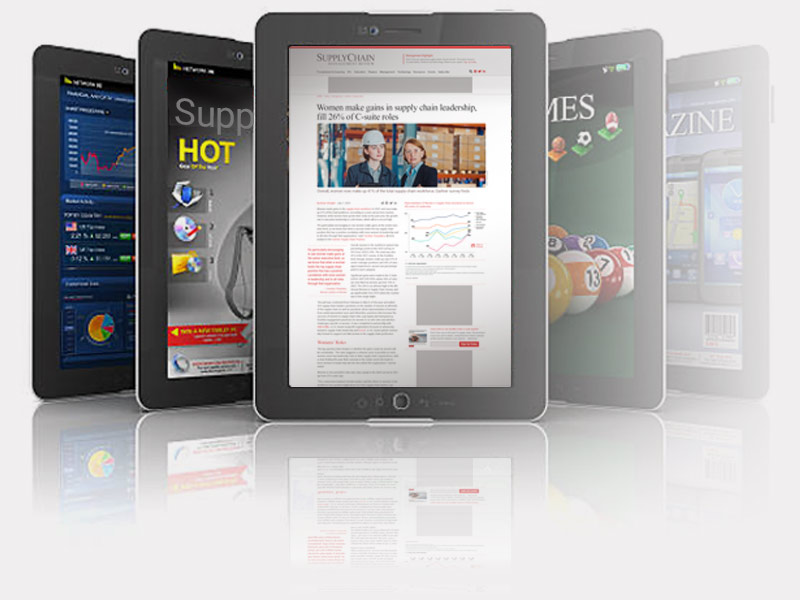
Editors’ Picks



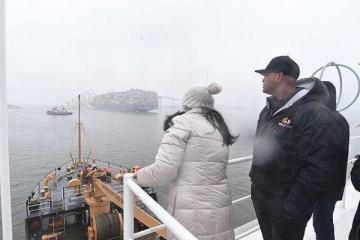