Editor’s Note: Every year, 40 or so students in the MIT Center for Transportation & Logistics' (MIT CTL) Master of Supply Chain Management (SCM) program complete one-year research projects. The students are early-career business professionals from multiple countries with 2 to 10 years of experience in the industry. Most of the research projects are chosen, sponsored by, and carried out in collaboration with multinational corporations. Joint teams that include MIT SCM students and MIT CTL faculty work on the real-world problems. In this series, we summarize a selection of the latest SCM research.
On-shelf availability (OSA) is a key metric in the consumer-packaged goods (CPG) industry. In many cases, out of stock (OOS) situations can lead to loss of sales. Working with a global CPG company, researchers at MIT's Center for Transportation and Logistics developed a simple but effective method to identify, aggregate and analyze OOS patterns in order to gain meaningful insights into ways to improve OSA.
Background and problems
The CPG company manufactures products, stores finished goods mainly in consolidation centers, and ships to large retailers across the US. Shipments typically go to retailers' distribution centers (DCs), and the products are distributed to each store from there.
The problem investigated by the researchers is that the manufacturer's retailer customers were experiencing frequent OOS events at their DCs. The company believes that inventory drops follow a pattern that might offer insights into how to reduce the number of out of stock events. If there is a sudden inventory drop in the two days prior to an OOS, then actions could be taken to replenish inventory in the DCs and hence to minimize the impact of an OOS outcome.
One product line was studied for the project. Due to the complexity of the data structure and data volume, 20 representative SKUs were selected and further analyzed. Each SKU contains inventory on-hand information for all 42 DCs, with each DC providing one year's worth of data points. It would be nearly impossible to manually go through each DC for each SKU to detect a series of potential patterns. Moreover, there are 432 unique SKUs in the given product family. The range of inventory value is between 0 and 600, which makes pattern recognition even harder.
Pattern recognition
The approach taken by the researchers is to normalize a time-series dataset, search for similar OOS patterns, and analyze particular patterns. By focusing on a specific type of pattern in which an OOS event happens within a pre-defined time period, it was possible to detect some interesting behaviors. First, as the time period is decreased (from 7 days to 4 days) the associated OOS pattern happens more frequently. Second, a steep inventory drop appears to be an infrequent event, based on the sample dataset.
These observations yielded some potential insights. For example, stock-outs don't seem to be predictable based solely on DC data, owing to the low frequency of steep drops in inventory.
Simple, effective analysis
Using the index and similarity search methods, a large-scale series of OOS patterns can be identified and aggregated. Instead of manually going through each time series, a pattern can be generalized and described using both the index and the absolute inventory value. It takes about 20 minutes to search for several patterns for one SKU. Essentially, the CPG firm could use this method to test more potential patterns and SKUs and then aggregate by SKU-DC combinations. This method could possibly be scaled to all 432 SKUs to aggregate patterns from 4 million transactions, with an output of small sets of repeated patterns.
Using the identified pattern dataset, retail store data could be further explored to connect point of sale data and OOS outcomes and identify the drivers of out of stocks.
The SCM research project Improving Analyzing Out of Stock Patterns for a Consumer Goods Company was authored by Xu (Tony) Li and supervised by Jim Rice, Deputy Director, MIT CTL ([email protected]), and Dr. Sergio Caballero, Research Scientist, MIT CTL ([email protected]), For more information on the research please contact Jim Rice or Sergio Caballero.
SC
MR
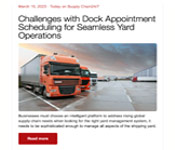
Latest Supply Chain News
- Technology’s role in mending supply chain fragility after recent disruptions
- Tech investments bring revenue increases, survey finds
- Survey reveals strategies for addressing supply chain, logistics labor shortages
- Israel, Ukraine aid package to increase pressure on aerospace and defense supply chains
- How CPG brands can deliver on supplier diversity promises
- More News
Latest Podcast
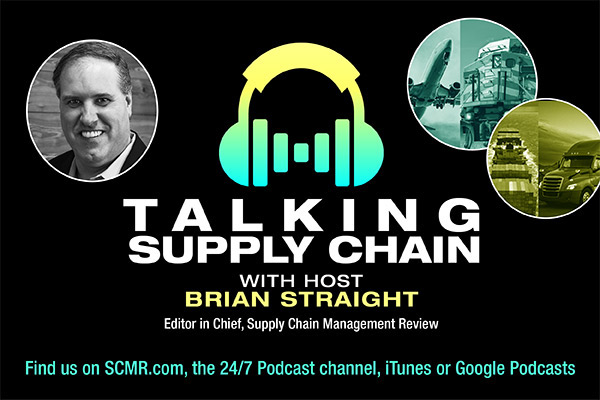
Explore
Topics
The Academy News
- AI, virtual reality is bringing experiential learning into the modern age
- Predicting stockouts: Enhancing FMCG resilience through data-driven insights
- Finding the Right Approach for Supply Chain Education
- The Supply Chain Triad
- Innovating Supply Chain Higher Education with Generative AI
- How Smart Supply Chain Management Boosts Brand Identity
- More The Academy
Latest Academy Resources
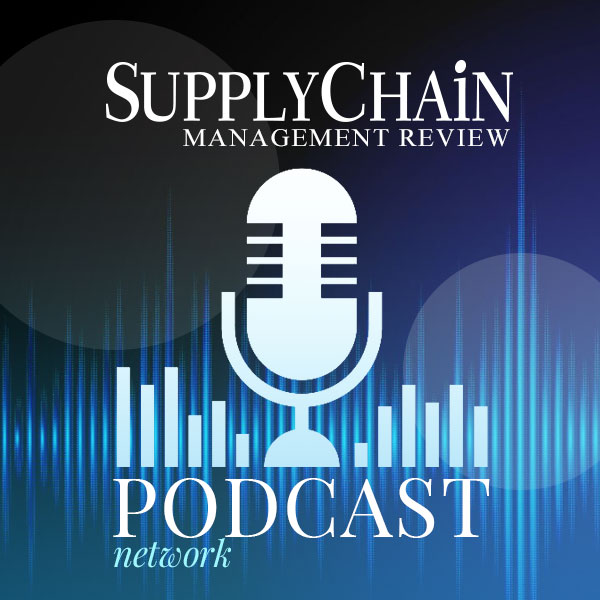
Subscribe
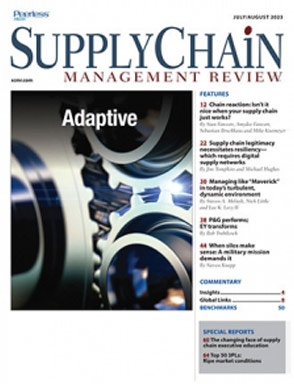
Supply Chain Management Review delivers the best industry content.
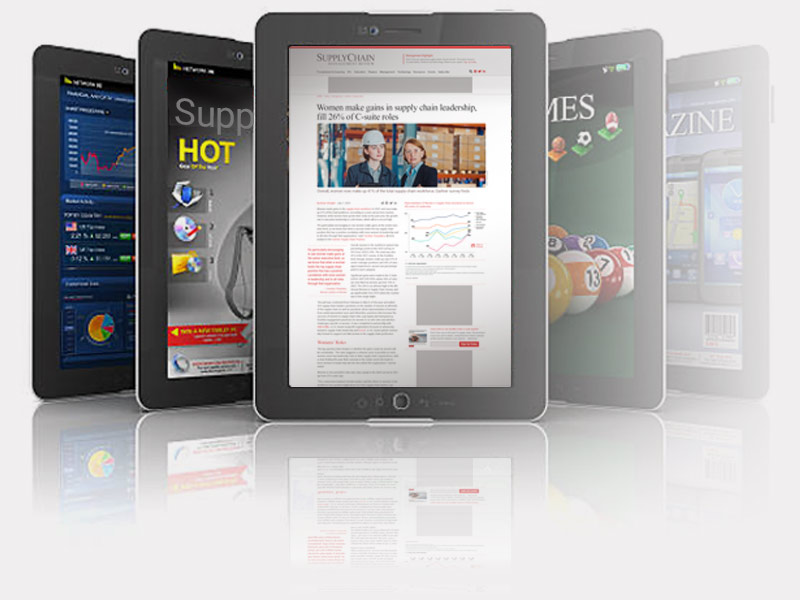
Editors’ Picks
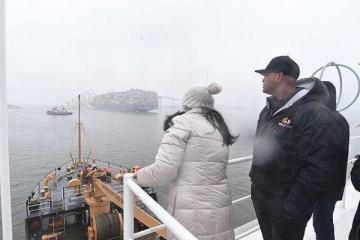

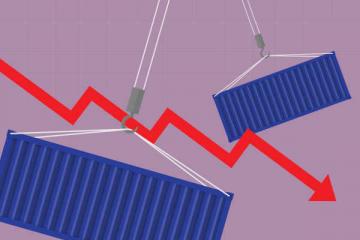
